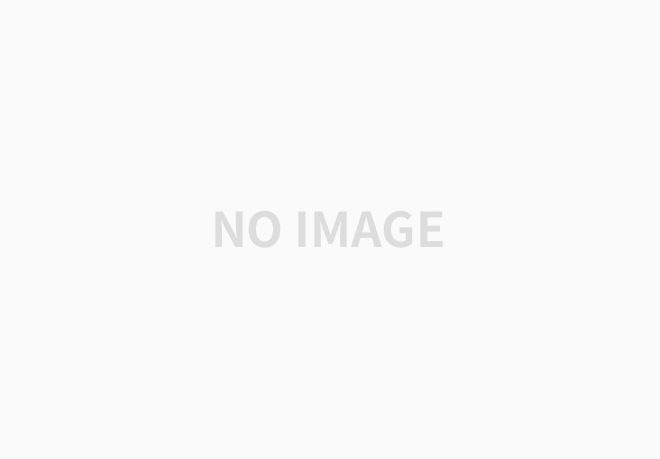
안녕하세요, HELLO
머신러닝, 딥러닝 분야는 좋은 성능의 모델을 만들기 위한 많은 시간, 컴퓨팅 파워, 인력 등이 필요한 '시행착오 (Trial and error)'가 많이 필요합니다. 이러한 반복적이고, 실험적인 모델의 학습을 개선하기 위해 최근에는 데이터를 입력하면 사람의 많은 개입(Human Assistance) 없이 높은 성능의 모델을 만들어내는 automated machine learning, 이른바 AutoML 분야가 각광받고 있습니다.
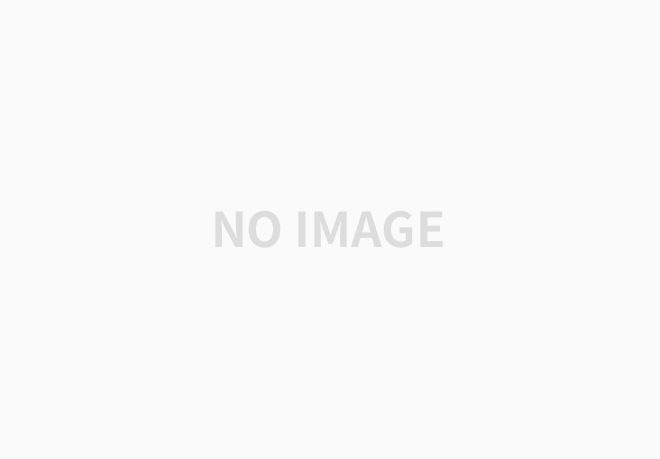
AutoML 분야는 데이터, 변수, 모델의 관점에서 data preparation, feature engineering 그리고 model generation, model evaluation 이렇게 4개 분야로 정리하고 있습니다. (ref. AutoML: A Survey of the State-of-the-Art, 2021)
각 분야가 광대하며, 내용이 깊기에 AutoML NAS (Neural Architecture Search)에서 최신 트렌드 논문을 선택해서 스터디를 진행하게 되었습니다. (PAPER LIST : URL) Linkedin에도 업데이트를 진행하고 있습니다.
이번에 살펴본 "AutoML-Zero: Evolving Machine Learning Algorithms From Scratch"논문은 저명한 학술지 Nature Machine Intelligence에 게재되었습니다. 이 논문은 적응성과 효율성 측면에서 기존 방법을 능가하는, 사람의 개입 없이 자동으로 머신러닝 알고리즘을 발견하고 최적화할 수 있는 새로운 알고리즘을 제시합니다.
논문 저자인 Google Brain의 Esteban Real, Chen Liang, and Quoc V. Le는 다양한 기본 연산 세트를 사용해 머신러닝 알고리즘을 처음부터 진화시키는 진화적 검색 기반 접근법을 개발했습니다. 사전 정의된 템플릿이나 빌딩 블록에 의존하는 기존의 AutoML 방법과 달리, AutoML-Zero는 다양한 작업을 해결하기 위해 새로운 학습 규칙을 적용하고 생성할 수 있는 다용도 알고리즘입니다.
이 방법론은 유전자 프로그래밍과 돌연변이 연산을 사용하여 잠재적인 알고리즘의 공간을 탐색함으로써 복잡한 문제를 해결할 수 있는 새롭고 효율적인 학습 규칙을 발견할 수 있게 합니다. AutoML-Zero는 이미지 분류, 강화 학습, 자연어 처리 등 다양하고 까다로운 작업에서 인상적인 결과를 입증했습니다. 이러한 놀라운 적응성과 새로운 학습 알고리즘을 발견할 수 있는 능력을 갖춘 AutoML-Zero는 머신러닝에 접근하는 방식을 혁신하고 컴퓨터 비전, 로봇 공학, 자연어 이해와 같은 분야의 발전을 가속화할 수 있는 잠재력을 가지고 있습니다.
I recently came across an innovative paper in the field of automated machine learning, titled "AutoML-Zero: Evolving Machine Learning Algorithms From Scratch." Published in the prestigious journal Nature Machine Intelligence, this paper presents a novel algorithm that can automatically discover and optimize machine learning algorithms without any human intervention, surpassing existing methods in terms of adaptability and efficiency.
The authors, Esteban Real, Chen Liang, and Quoc V. Le from Google Brain, developed an evolutionary search-based approach that evolves machine learning algorithms from scratch using a diverse set of primitive operations. Unlike traditional AutoML methods that rely on pre-defined templates or building blocks, AutoML-Zero is a versatile algorithm that can adapt and create new learning rules to solve different tasks.
Their methodology employs genetic programming and mutation operations to explore the space of potential algorithms, enabling the discovery of novel and efficient learning rules that can tackle complex problems. AutoML-Zero has demonstrated impressive results on a variety of challenging tasks, including image classification, reinforcement learning, and natural language processing.
This paper marks a significant milestone in the field of automated machine learning and carries profound implications for researchers working on artificial intelligence. With its remarkable adaptability and capacity for discovering new learning algorithms, AutoML-Zero has the potential to revolutionize the way we approach machine learning and accelerate advancements in domains such as computer vision, robotics, and natural language understanding.
■ ORIGINAL PAPER
■ PAPER REVIEW
■ 마무리
'AutoML-Zero: Evolving Machine Learning Algorithms From Scratch'에 대해서 알아봤습니다.
좋아요와 댓글 부탁드리며,
오늘 하루도 즐거운 날 되시길 기도하겠습니다 :)
댓글