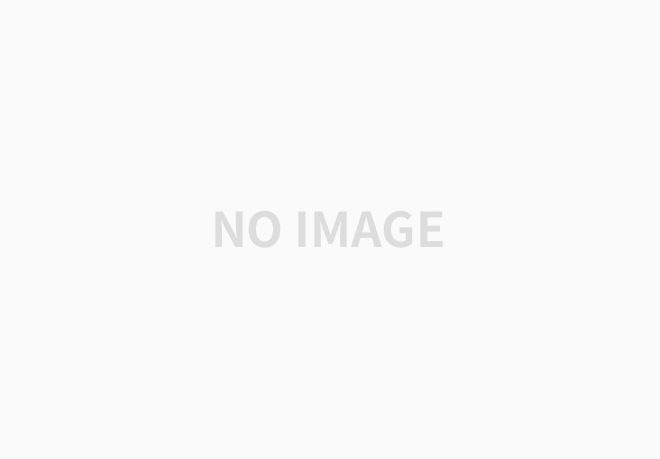
안녕하세요, HELLO
오늘은 DeepLearning.AI, Amazon Web Services에서 진행하는 Practical Data Science Specialization의 두 번째 과정인 "Build, Train, and Deploy ML Pipelines using BERT"을 정리하려고 합니다.
"Build, Train, and Deploy ML Pipelines using BERT"의 강의를 통해 'Automate a natural language processing task by building an end-to-end machine learning pipeline'에 대해서 배우게 됩니다. 강의는 아래와 같이 구성되어 있습니다.
~ Feature Engineering and Feature Store
~ Train, Debug and Profile a Machine Learning Model
~ Deploy End-to-End Machine Learning pipelines
"Build, Train, and Deploy ML Pipelines using BERT"의 2주차 "Train, Debug and Profile a Machine Learning Model"의 강의 내용입니다.
CHAPTER 1. 'Training of a custom model'
CHAPTER 2. 'Training of a custom model with Amazon SageMaker'
CHAPTER 1. 'Training of a custom model'
□ Pre-trained models
NLP 모델을 훈련하는 것은 많은 컴퓨팅 파워와 시간을 소요합니다. 이에 따라 Hugging Face, pytorch에서 사전에 NLP 모델을 훈련시킨 pre-trained model이 많습니다.
그리고 pre-trained model을 바탕으로 훈련 시간을 줄일 수 있습니다.
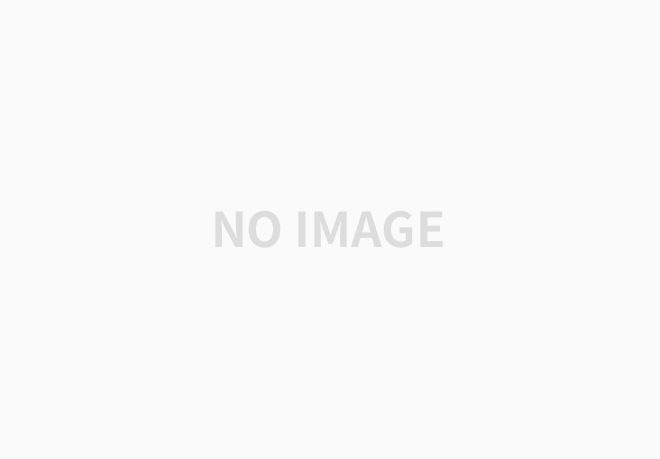
Pre-trained data에서 Fine-Tuning은 pre-trained data에 훈련에 사용할 데이터를 이어서 훈련시키는 것을 의미합니다. 예를 들어, 상품 리뷰 분석 모델을 만들고 싶은 경우에, 기존에 훈련된 pre-trained model에 상품 리뷰 text를 훈련시키는 것입니다.
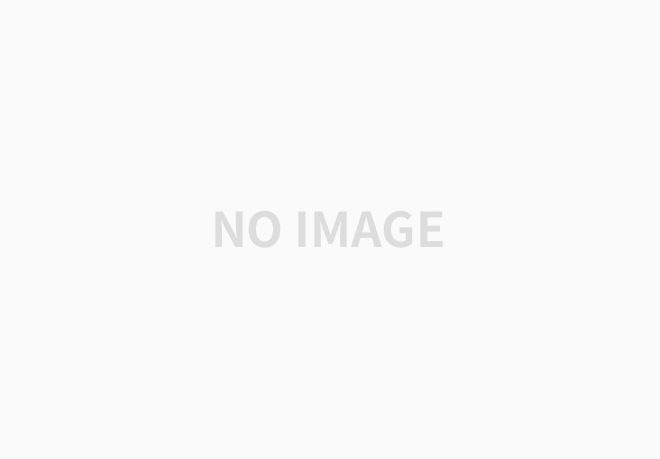
다양한 pre-trained model이 open source로 공개되어, 이를 활용할 수 있습니다.
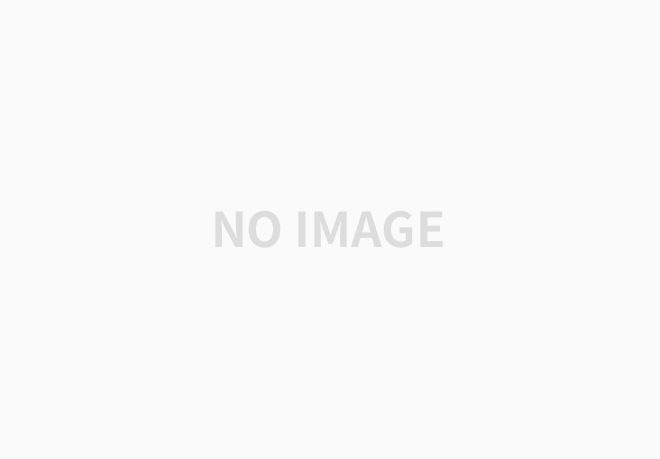
또한 AWS에 위의 pre-trained model을 직접 연결해서 활용할 수 있습니다.
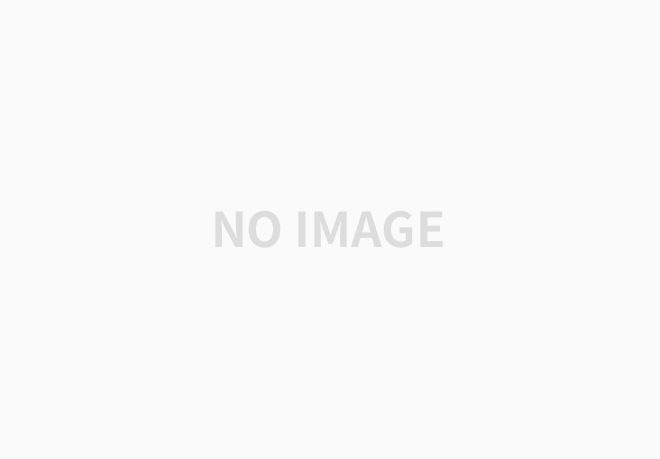
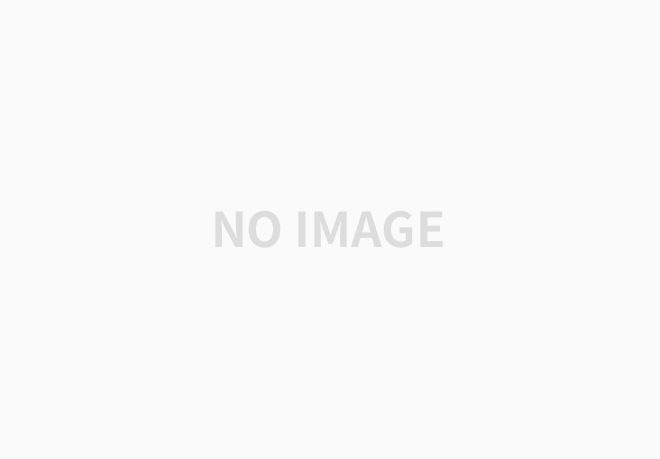
□ Pre-trained BERT models
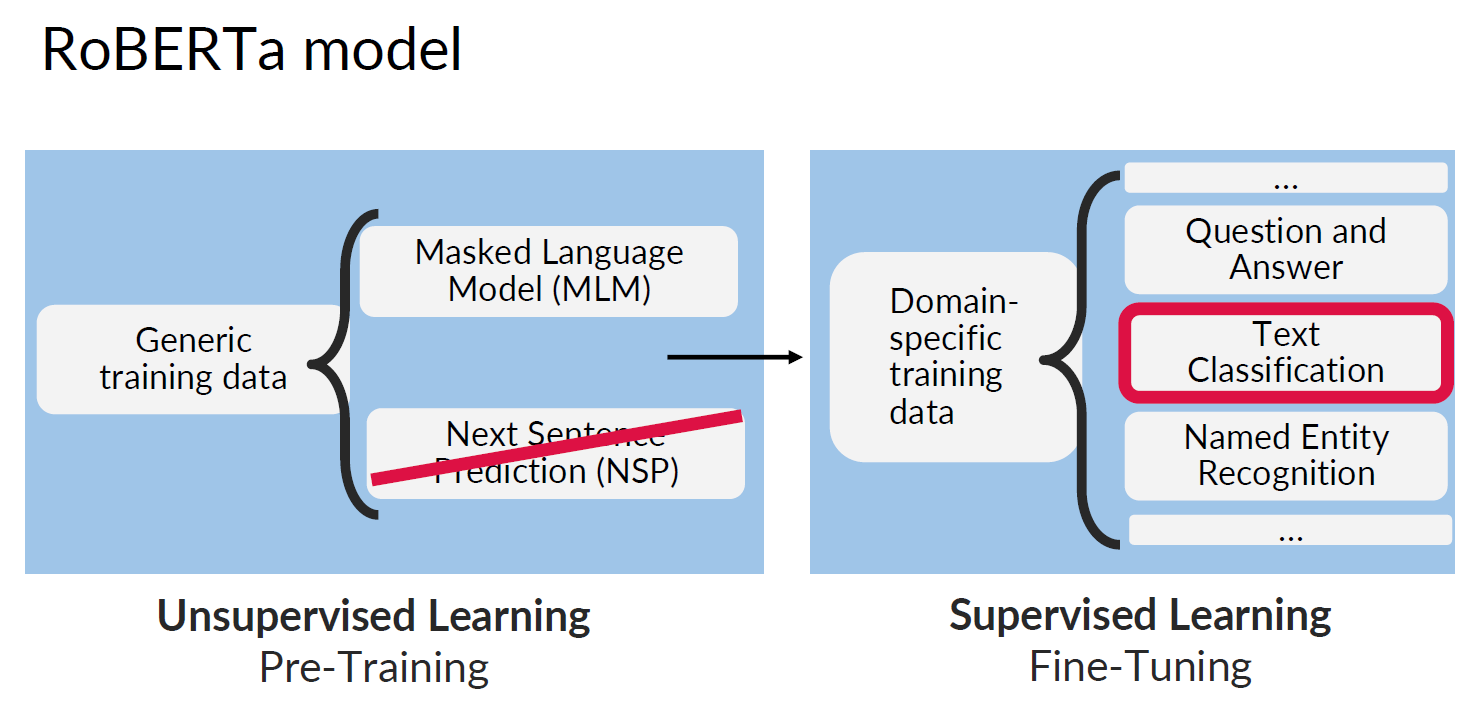
BERT는 word-masking(MLM)과 next-sentence prediction(NSP)을 진행합니다. MLM을 통해 단어를 masking, 가리며 이를 예측함으로써 weights를 업데이트합니다. 위 과정을 반복하여 훈련하며, 이후에 다음 문장을 예측합니다 (NSP).
CHAPTER 2. 'Training of a custom model with Amazon SageMaker'
□ Train a custom model with Amazon SageMaker
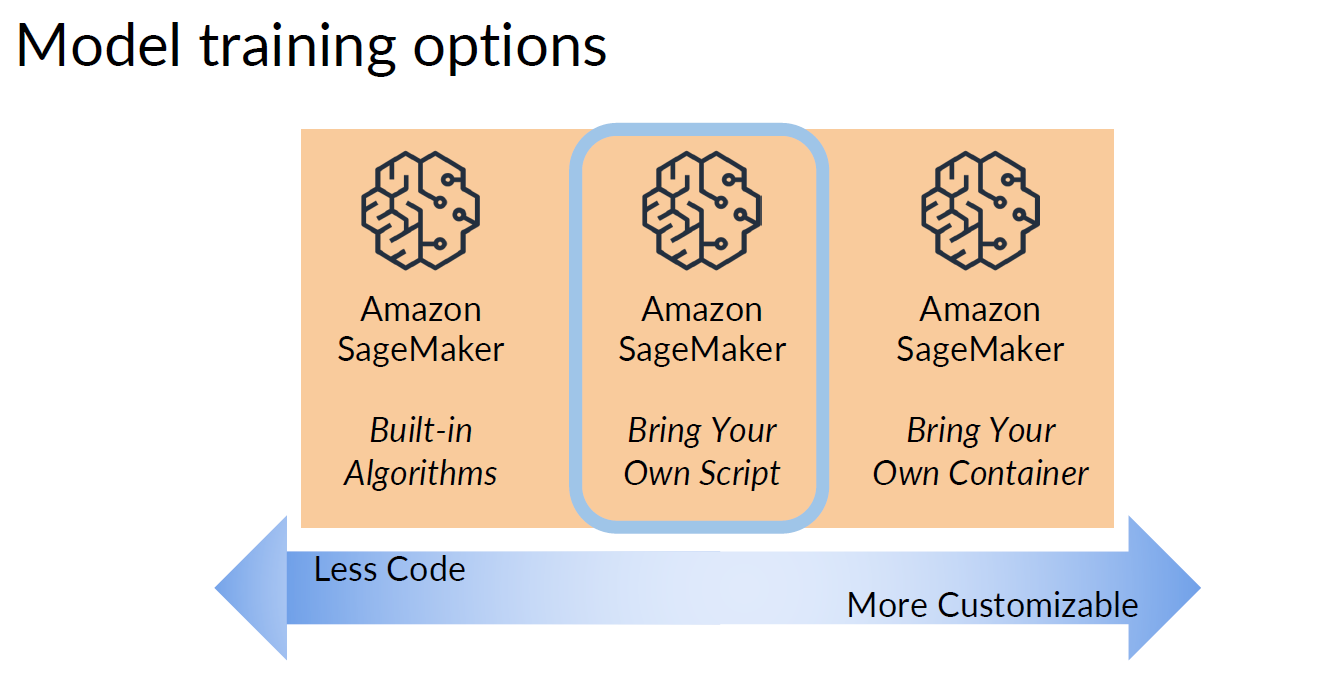
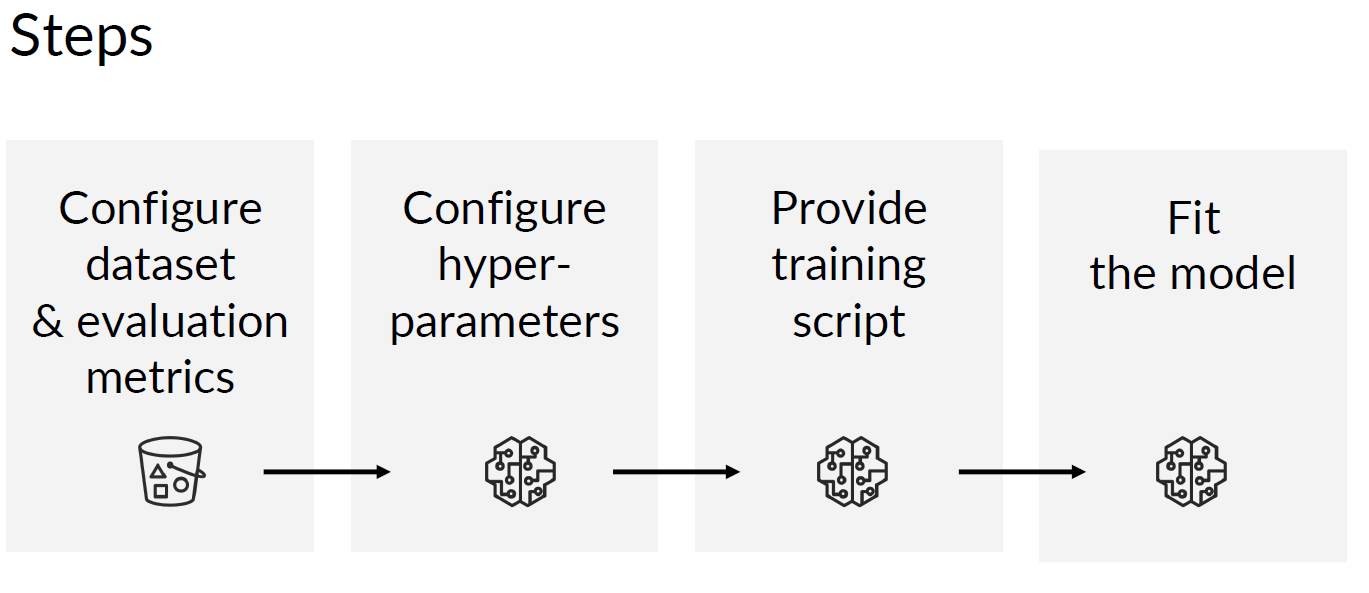
□ Debug and Profile Models with Amazon SageMaker Debugger
AWS에서 자동으로 모델의 training error를 확인할 수 있습니다.
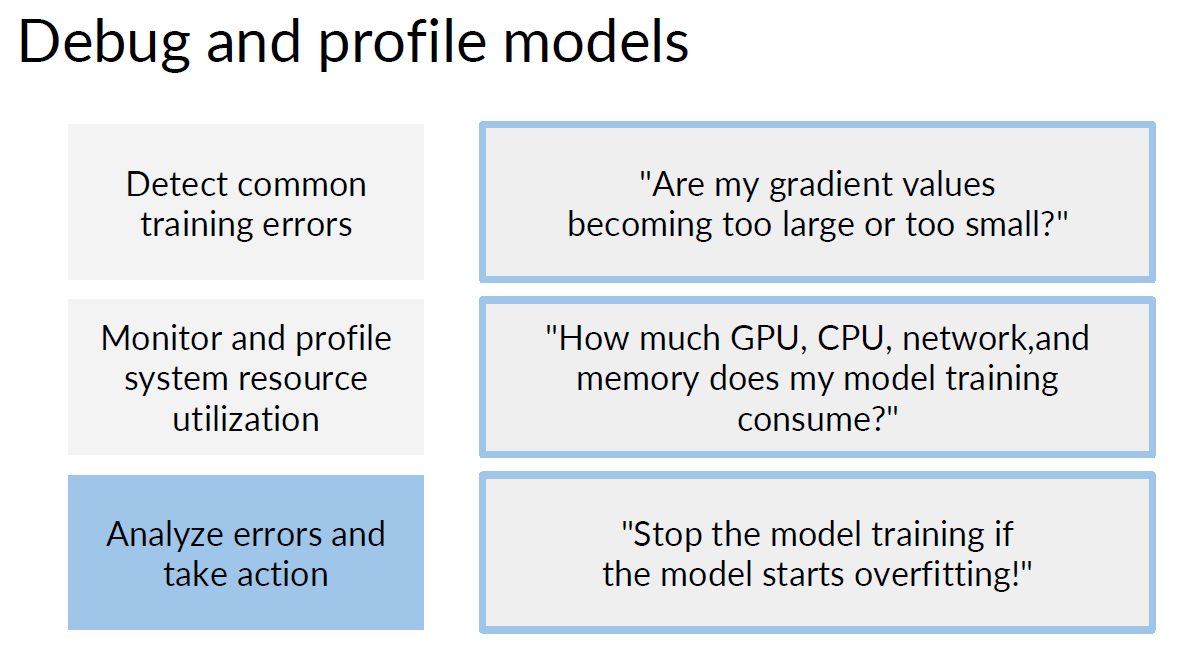
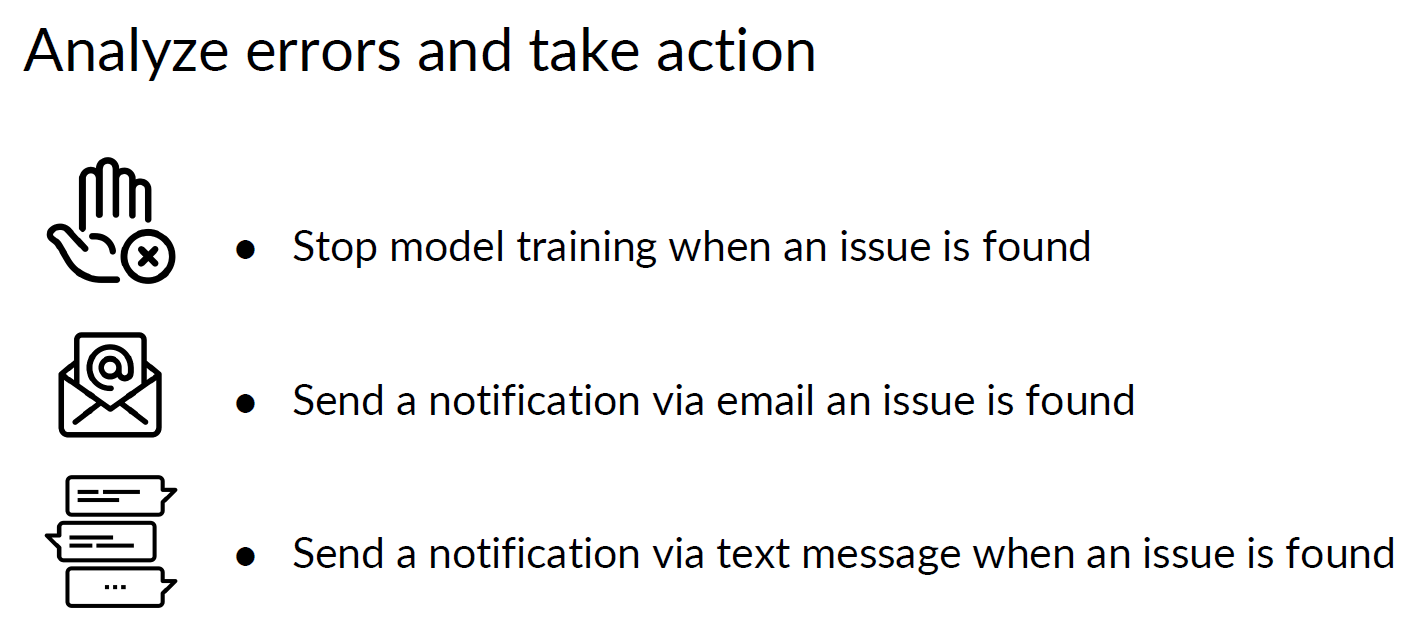
■ 마무리
"Build, Train, and Deploy ML Pipelines using BERT"의 2주차 "Train, Debug and Profile a Machine Learning Model"의 강의에 대해서 정리해봤습니다.
그럼 오늘 하루도 즐거운 나날 되길 기도하겠습니다
좋아요와 댓글 부탁드립니다 :)
감사합니다.
댓글